Master's Thesis
Threshold response models applied to best-of-n in Swarm Robotics
Swarm robotics has emerged as a critical research area, particularly inspired by the collective behaviours and decentralized decision-making processes observed in social insects such as ants, and bees. This research aims to take inspiration from such natural social networks to offer a comprehensive exploration of threshold response models applied to the best-of-n problem in swarm robotics. The study builds upon existing theories and frameworks discussed in the literature, including natural swarm behaviours and decentralized control mechanisms, to offer a more balanced and effective approach to swarms that can choose from n>2 options. To validate these theoretical constructs, the research employs a rigorous simulation-based methodology. Various environmental conditions and constraints were considered to test the adaptability and resilience of the proposed models. The findings indicate significant improvements without compromising the quality of the decisions made by the swarm. In-depth discussions of these results reveal additional insights, such as the impact of threshold sensitivity on decision quality and the scalability challenges associated with an increasing number of options. While the study provides robust evidence in support of the proposed models, it also acknowledges limitations, primarily the reliance on simulation data. As such, future research directions include empirical validation using physical robot swarms and the fine-tuning of model parameters to adapt to specific environmental conditions. Overall, this dissertation serves as a contribution to the field of swarm robotics, offering a theoretically grounded, robust, and efficient model for decentralized decision-making in complex, dynamic settings.
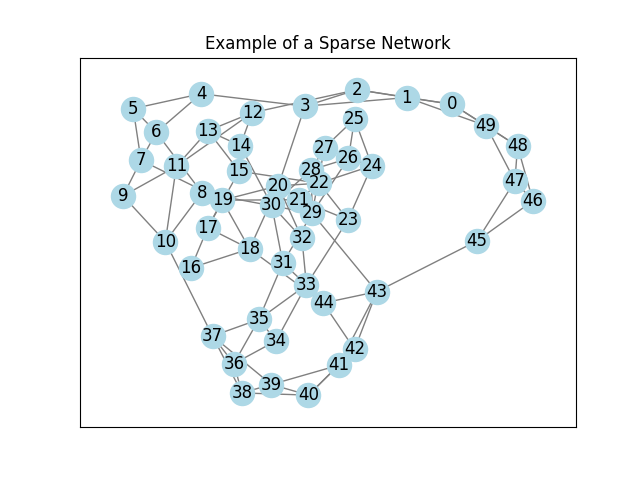